Computational physics for modelling complex scanning probe images
Sophisticated machine learning software shows potential for establishing semi-autonomous SPM systems
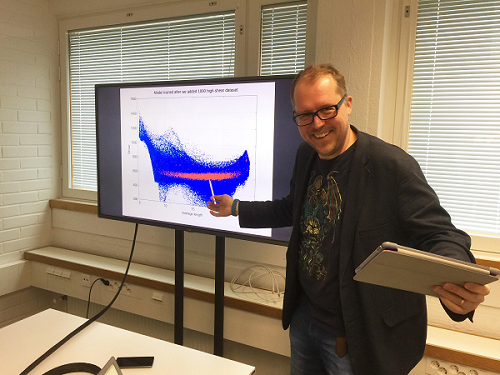
Adam Foster, Professor, Nano Life Sciences Institute (WPI-NanoLSI), Kanazawa University
Adam Foster is an overseas PI at the WPI Nano Life Science Institute (WPI-NanoLSI) and leader of the Surface and Interfaces at the Nanoscale at Aalto University in Finland. Foster is a computational physicist applying his expertise in first principles simulations to a wide range of areas including self-assembly of materials, solid-liquid interfaces, often in conjunction with scanning probe microscopy research.
“I generally working at the atomistic level,” explains Foster. “I look from both the quantum and angle classical viewpoints and work closely with experimentalists. This is what led to my work with the NanoLSI. They are world leaders in scanning probe microscopy with many fascinating images to analyze.”
Effects of COVID-19 on research activities in Finland
“Finland reacted very quickly, so for two months there were restrictions, and we could not go out,” recalls Foster. “It’s much better than certain other countries. My group does not require experimental equipment, and we adapted very quickly to the new norm. But initially, it was difficult for experimental groups because they could not access their instrumentation. But now, in February 2021, it is possible to pursue experimental research by following the university’s rules for social distancing, tracking, and booking facilities.” Interestingly, in December 2020 Foster noticed an increase in his citations that he thinks is because many more people had time to write papers due to lockdowns. “Colleagues working in my field of research have also seen similar increases in citations of their publications.”
Research with colleagues at the NanoLSI
Foster is a member of the Computational Science group at NanoLSI and using his expertise to analyze scanning probe images including those of complex biological systems. “One of our goals is to develop biological models to reproduce experimental results,” explains Foster. “For example, we ask ourselves that when measuring the force of penetration of a probe through a cell membrane, can we vary parameters in our models so that experimentalists can see the same things, and what do the results tell us about the state of health of cells.”
Notably, Foster has developed a range of machine learning tools to analyze SPM images, with examples including rapid pattern analysis of the adhesion properties of healthy and cancerous cells using images taken with scanning probes. “The massive data derived from modern high speed and ultra-high resolution SPM experiments has reached a stage where conventional atomistic modeling cannot cope,” says Foster. “This is where machine learning becomes important for analyzing huge amounts of data on the atomic scale. We have been successful in doing this in biological systems, where the signal is noisy and messy.”
The future: Machine learning and autonomous scanning probe systems
Foster and his colleagues are working on the development of modelling methodology to aid experimentalists during in-situ measurements. “Ultimately I envisage experimentalists seeing real time images of their samples with quantitative information on specific molecules and atoms being observed,” explains Foster. “But this is a complicated system, with components that include ionic aqueous solutions, functionalized probes, and of course the actual biology of the system.”
Foster says that the ultimate extension of this approach to imaging will be autonomous systems that do not require experimentalists. “We first replace ourselves as modelers, and next autonomous systems will not need experimentalists, perhaps!”
Foster is looking forward to visiting Kanazawa again once restrictions are lifted. “I want to catch up with my colleagues at the NanoLSI on developments in scanning probe microscopy as well as visit some of my favorite fish and sushi restaurants in the City.”
Research highlights
In their paper entitled “Automated structure discovery in atomic force microscopy”, Foster and his colleagues describe the development of a deep learning systematic software approach to analyze low temperature AFM images of 1S-camphor on Cu (111) and directly predict their molecular structure [1]. This approach could potentially enable routine high resolution AFM measurements of otherwise surfaces such as nonplanar molecules.
Software and machine learning is also the topic of another paper, where Foster and colleagues describe power of the ‘DScribe’ software package for machine learning [2].The software was used to predict formation energy for solids and ionic charge in organic molecules.
In Nanoscale, Foster and an international collaboration of scientists including Takeshi Fukuma of the NanoLSI, describe how the scanning force microscopy images of calcite-water interfaces are affected by the probes used to obtain the images [3]. Comparison of experimentally scanned images and simulations yielded detailed information about the structure and properties the tips used for the experiments.
References
[1] Benjamin Alldritt et al, Automated structure discovery in atomic force microscopy, Science Advances 26 Feb 2020: Vol. 6, no. 9, eaay6913. DOI: 10.1126/sciadv.aay6913
https://advances.sciencemag.org/content/6/9/eaay6913
[2] Lauri Himanen et al, DScribe: Library of descriptors for machine learning in materials science, Computer Physics Communications, 247, 106949, (2020). https://doi.org/10.1016/j.cpc.2019.106949
[3] Keisuke Miyazawa et al, Tip dependence of three-dimensional scanning force microscopy images of calcite–water interfaces investigated by simulation and experiments, Nanoscale, first published on 10 June 2020, https://doi.org/10.1039/D0NR02043E
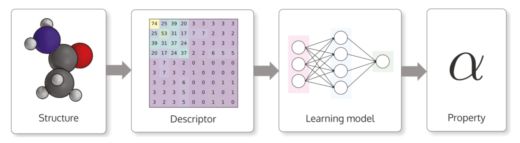
Typical workflow for making machine learning based materials property predictions for atomistic structures. An atomic structure is transformed into a numerical representation called a descriptor. This descriptor is then used as an input for a machine learning model that is trained to output a property for the structure.
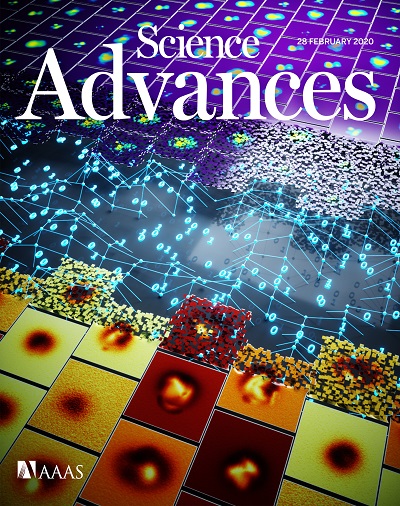
Experimental Atomic Force Microscopy images are converted into image descriptors using a machine learning network, providing a signature that can be used in molecular identification.
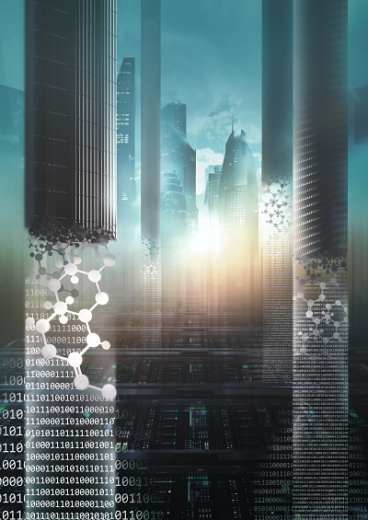
Vision of future materials research build on data and machine learning.
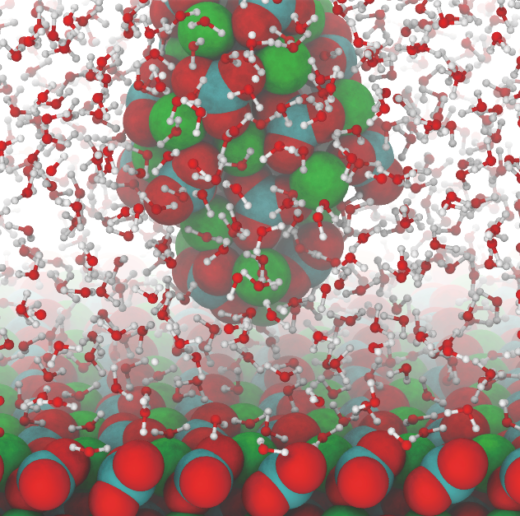
Atomistic snapshot from simulations of an Atomic Force Microscopy tip imaging calcite in water.
Further information
NanoLSI Podcasts
This episode offers greater insights into Professor Adam Foster’s research at the NanoLSI.